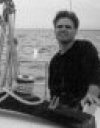
randallmayes
Submitted by randallmayes on

Rebooting AI: Book Review
If you follow AI, you have probably read about what happened when chess and Go masters were matched against AI using deep learning. To put it politely, AI humbled the mere mortals. Yann LeCun, a professor at NYU and a scientist affiliated with Facebook, recently co-shared the Turing Award (1 million USD) for his role in the development of deep learning.
Meanwhile, the same campus houses Professor Emeritus Gary Marcus. Ironically, he has become the mouthpiece for popularizing the limitations of deep learning. Somewhat unique as an AI researcher, Marcus brings the perspective of a psychologist and neuroscientist having studied under Steven Pinker at MIT. In the transition from an idea to a pioneering transportation company, Uber recruited several dozen employees from Carnegie Mellon’s AI department and purchased his start-up Geometric Intelligence. His new start-up Robust.AI focuses on robotics.
Marcus also has side gig writing about AI for The New Yorker and The New York Times and has authored several books on a diverse variety of AI related topics. On September 10, 2019 Pantheon/Knopf Doubleday will release Rebooting AI: Building Artificial Intelligence We Can Trust by Marcus and another NYU professor, Ernest Davis, a machine intelligence and reasoning specialist.
Rebooting AI is a continuation of the theme of the limitations of deep learning and how to proceed forward. This is a timely topic since AI is at a pivotal point in its history. Deep learning is a branch of machine learning and the engine behind the second wave of AI. AI is now entering its third wave, which aims to address the limitations of deep learning and AI in general.
AI’s Roller Coaster Ride
For technology analysts like myself, Marcus’ works are a treasure trove of information for forecasting the future development of AI. When plotting the past progress of various emerging technologies over time, historically rather than a straight line, the pattern resembles an S-curve. However, AI’s past progress more closely resembles a roller coaster ride than an S-curve.
Following World War II, the United States continued to develop military technologies in order to keep the American military prepared during the Cold War. In response to the Russian satellite Sputnik, in 1958 President Dwight Eisenhower established what is now called the Defense Advanced Research Projects Agency (DARPA) to prevent losing the technological edge to foreign states which could blindside the United States. As AI became a discipline, DARPA funded research in programs at MIT’s AI Lab, Stanford Research Institute (SRI), the Carnegie Institute of Technology (now Carnegie Mellon University), and the RAND Corporation for the development of expert systems.
Expert systems drove the first wave of AI which dates back to the 1950s and continued into the early 1990s. It is characterized by hardware that utilizes knowledge from experts in specific domains. In contrast to deep learning, expert systems are rules based (if, then) rather than using software for analyzing data. If you have ever completed your federal income taxes online, you have experienced first wave AI.
From 1974-1980, expert systems experienced an AI Winter, a period of reduced general interest and funding. AI researchers had underestimated human brain complexity and overhyped AI’s capabilities. Then in 1980, the commercial adoption of expert systems and the Japanese Fifth Generation Computer project which introduced parallel processing—another Sputnik moment for the United States—revived AI. However, a drawback of expert systems is the infinite number of if, then rules. Then in 1989, desktop computers began replacing mainframes and a shift from knowledge based to data driven systems leading to a second AI Winter.
In the 1990s, machine learning enabled by increased computer speed and processing power, better algorithms, and the ability to capture and store massive amounts of data spurred the second wave of AI. Using neural networks, scientists begin to create programs that analyze large quantities of data to detect patterns and develop predictions. In 2006, business began using the enhanced version of machine learning known as deep learning which has multiple layers of nodes in the neural networks.
A Third AI Winter?
Some AI experts including the authors believe that deep learning may have too many limitations to develop the field much further. The list of limitations is extensive and present formidable challenges for machines to equal human intelligence rather than augment it.
1) Human brain complexity
The authors point out that machines can beat former world champion Garry Kasparov at chess, but they still have problems turning door knobs. For applications in self-driving cars, the ability to distinguish between objects, and to distinguish those from pictures of the objects, is necessary. Currently, it requires hundreds of thousands of expensive labeled data sets to achieve high accuracy rates. These are tasks that a 10 year old child can perform easily, a phenomenon known as Moravec’s paradox.
While deep learning is patterned after cognitive tasks using the neocortex, neuroscientists estimate that a quarter of the brain evolved to perform more basic skills. How to provide machines with common sense and reasoning ability is a challenge for AI researchers.
The chapter titled Insights into the Human Mind draws on Marcus’ background in the fields of cognitive psychology, cognitive development, and linguistics which impact human intelligence. Human intelligence is a combination of innate and learned skills, while deep learning intelligence is strictly learned. “Since babies seem to be built with some understanding of objects and other agents,” Marcus suggests, “we should think hard about how to build AIs with similarly rich starting points.”
2) The black box
The deep learning systems that beat masters at board games are not taught by humans, rather are learned by the machines on their own through millions of games. Not only can humans not benefit by learning from the machines, algorithms used to make decisions may contain hidden biases from the programmers.
3) Narrow AI
While humans have general intelligence, currently machine intelligence has a limited scope.
4) The transfer problem
Each task has unique data and does not apply to other tasks. For example, in each basketball game in a NCAA bracket, you have to start from scratch with new data and generate new predictions.
5) Cause and effect
Similar to humans analyzing data sets, deep learning algorithms reveal correlation, not causality.
With all the hurdles emerging technologies encounter, it is easy to understand why S-curves have acquired the nickname of hype cycles. Marcus fears that overhyping AI may result in another AI winter. Fortunately, even with all the current limitations the field is not moving towards that scenario.
The Chinese government and Silicon Valley firms are investing billions of dollars on developing AI applications, alternative forms of AI, and hybrid methods. The Allen Institute of Brain Sciences and the American and European governments have invested billions of dollars on programs to better understand how the human brain works. Also, DARPA launched a $2 billion program called the AI Next Campaign to overcome the current limitations of deep learning. DARPA envisions the third wave of AI as one focusing on contextual adaptation, explainability, and common sense reasoning.
The Third Wave of AI will determine if we experience a third AI winter or machine intelligence similar to humans. Most AI experts now believe that if the singularity does occur, it will take several more decades. If the Third Wave of AI is developed correctly, humans will have more prosperous and easier lives. If developed incorrectly, humans could potentially face a robot takeover with mass unemployment and the terminator scenario where AI harms humans.
Sources
DARPA. "AI Next Campaign."
https://www.darpa.mil/work-with-us/ai-next-campaign
DARPA. "DARPA Announces $2 Billion Campaign to Develop Next Wave of AI Technologies." September 7, 2018.
https://www.darpa.mil/news-events/2018-09-07
Marcus, Gary. "Deep Learning: A Critical Appraisal." ArXiv. January 2, 2017. 17-18.
https://arxiv.org/ftp/arxiv/papers/1801/1801.00631.pdf
E-mail correspondence from Gary Marcus on September 3, 2019.
Randall Mayes is a technology analyst, an instructor in Duke University’s OLLI Program specializing in emerging technologies including AI, smart manufacturing, and synthetic biology, and author. His new book, Trade-Offs: A Handbook for the Sixth Revolution, is forthcoming.
Edited 10-16-2019 to correct Yann LeCun's affiliation.